Toolpath-Level Machine Monitoring Improves Aerospace OEE
Machine monitoring software that records historical efficiency to the toolpath level helped aerospace manufacturer Leesta improve its OEE by ten points.
Share




Hwacheon Machinery America, Inc.
Featured Content
View More



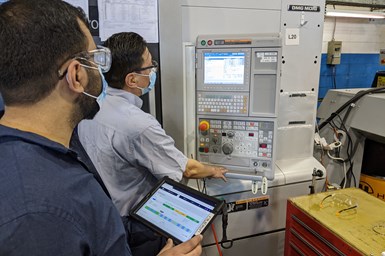
Leesta runs many repeat parts for its aerospace clients, so even improvements of a few seconds on its typical 10-30 minute cycle times add up quickly. Photo courtesy of JITbase.
Leesta Industries develops complex, high-precision components for the aerospace and defense markets, with a special emphasis on jet engine and landing gear components. The company’s lathes and four- to eight-axis CNC machines grant it wide-ranging capabilities, but inefficient programs hampered productivity. Machine monitoring software JITbase OPS revealed opportunities to reduce cycle times, extend lights-out periods and improve toolpath efficiency, all of which contributed to a rise in the company’s overall equipment efficiency (OEE).
Ernest Staub, Leesta’s president, credits these optimizations to toolpath-level production feedback that helped identify recurring spring passes and unnecessary planned stops.
The End of Spring (Passes)
Spring passes are repeat finishing cuts that eliminate leftover material. While the odd spring pass may be necessary, recurring spring passes can balloon program cycle times.
At Leesta, a toolpath-by-toolpath calculation of performance rates (actual times versus estimated times) confirmed which programs had low performance rates, with further investigation revealing that these programs often included recurring spring passes.
Specifically, the software automatically recognized that 51% of the overtime consisted of rework, or repeated portions of programs. Investigating the toolpaths flagged as the worst offenders (with low performance rates and many instances of rework) helped Leesta isolate and optimize these program sections by reducing speeds, targeting lower material removal per toolpath and including a planned spring pass automatically in the CNC program. The former two changes improved the quality of the initial pass, removing the need for shopfloor personnel to implement unplanned spring passes. Accounting for a spring pass improves the accuracy of Leesta’s cost, time and delivery estimates — especially useful for the manufacturer’s many repeat jobs. Together, the optimizations boosted program performance rates by nearly 50% in some cases.
JITbase’s OPS module and the M00 consolidations have set Leesta tracking toward a 10-point OEE gain in 2022.
Fewer Stops, Fewer Interventions
The software also identified planned stops (M00 commands) that lasted less than 30 seconds. Reasons for planned stops vary — performing inspections, removing chips, changing tools and changing part positions, to name a few. However, 30 seconds is generally too short a time to perform these manual operations, says JITbase president Frederic Scherer. Thus, these short stops are good signs of unnecessary M00 commands stemming from older program iterations or overcautious programming. M00 commands that are shorter than expected across multiple production cycles are also more likely to be unnecessary.
Leesta removed unnecessary M00 commands from more than 300 CNC milling and turning programs, including both roughing and finishing operations across a wide array of metals. These improvements shortened program cycle times by an average of 10% and reduced the number of planned stops by up to 67%. With fewer interventions, Leesta’s machines can run autonomously for longer, improving uptime and performance for a 4% gain in OEE.
Tracking Toward an Efficient Future
JITbase introduced its “CNC Program Optimizer” module — including the toolpath-level data analysis — during the last few months of 2021. Other new capabilities relate to the OPS (optimal path system), which Modern Machine Shop covered in June 2020. That version of OPS already tracked machine uptime and hands-on periods to help machinists determine which machines in their cell are the highest priority.
Now, the system includes a planning simulation tool that improves planners’ ability to visualize machinist workloads. Leesta plans to use this capability to visualize which programs can be run by the same machinist without leaving multiple machines down. If the real-time machine data reports any slowdowns on one machine, the software can then dynamically adjust machine allocation between personnel to maintain maximum uptime. Even before implementing this dynamic workflow, OPS and the M00 consolidations have set Leesta tracking toward a 10-point OEE gain in 2022.
Related Content
How to Mitigate Chatter to Boost Machining Rates
There are usually better solutions to chatter than just reducing the feed rate. Through vibration analysis, the chatter problem can be solved, enabling much higher metal removal rates, better quality and longer tool life.
Read MoreCutting Part Programming Times Through AI
CAM Assist cuts repetition from part programming — early users say it cuts tribal knowledge and could be a useful tool for training new programmers.
Read More6 Machine Shop Essentials to Stay Competitive
If you want to streamline production and be competitive in the industry, you will need far more than a standard three-axis CNC mill or two-axis CNC lathe and a few measuring tools.
Read MoreHow this Job Shop Grew Capacity Without Expanding Footprint
This shop relies on digital solutions to grow their manufacturing business. With this approach, W.A. Pfeiffer has achieved seamless end-to-end connectivity, shorter lead times and increased throughput.
Read MoreRead Next
Adding Part Traceability to CNC Machine Monitoring
Focusing on what makes money puts CNC machine monitoring data in context.
Read MoreMachine Monitoring Has a Lot to Learn
Supplementing CNC connections with sensors and machine learning enables predicting performance on the level of the individual part.
Read MoreMapping the Optimal Path for Machinists
Using data from machine monitoring systems and CNC programs, OPS calculates the most efficient path for operators to take across the shop floor, improving uptime and increasing machines per operator.
Read More